LCI Network
A quality life cycle assessment (LCA) depends on the availability and quality of life cycle inventory (LCI) data. LCA practitioners have been increasingly relying on dedicated LCI databases which provide LCI data for common unit processes of a wide range of products. Despite the convenience, these LCI databases still depend on time-consuming, expensive empirical data collection. Supported by a NSF CAREER grant, this project aims to transform the current practice of developing LCI databases into a faster, less expensive process that still generates reliable LCI data, by developing a computational and data-driven framework for estimating missing LCI data solely based on limited known LCI data, without relying on additional empirical data. It will (1) create a framework for modeling and analyzing LCI networks, (2) develop computational models for estimating missing LCI data, and (3) apply these models to evaluate LCI data quality and predict LCI data for emerging technologies.
Building upon the transformative promise seen from link prediction techniques in network science that enable the prediction of missing information of a network based on limited observations, this research develops computational and data-driven approaches as complementary alternatives for compiling LCI databases.
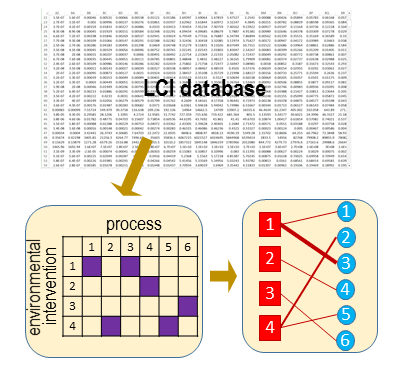